8 Real-World Machine Learning Use Cases Transforming Industries
Have you ever wondered how your smartphone knows what advertisements to show you or how your bank detects fraudulent transactions almost instantly? Machine learning (ML) isn’t just a buzzword; it’s a transformative technology redefining how businesses operate. Imagine peeking behind the curtain to see how these smart algorithms are applied across various industries to solve real problems and enhance our daily lives. This article unveils compelling ML use cases across different sectors, demonstrating its immense potential and real-world impact.
Use Case 1: Healthcare
In healthcare, ML is akin to a trusted doctor with a sixth sense, offering predictions and treatments that improve patient outcomes. The global AI in healthcare market, which includes ML applications, was valued at approximately USD 22.45 billion in 2023 and is projected to grow at a compound annual growth rate (CAGR) of 36.4% from 2024 to 2030. Let’s delve deeper into how ML is transforming this vital sector.
Key Applications:
Medical Imaging:
- Example: ML algorithms analyze medical images such as X-rays, MRIs, and CT scans. These advanced algorithms not only enhance the accuracy of disease detection but also significantly reduce the time required for diagnosis.
- Impact: Hospitals utilizing ML for medical imaging have reported faster and more accurate diagnoses, leading to improved patient outcomes.
Disease Diagnosis and Risk Prediction:
- Example: ML models assist in diagnosing diseases and predicting risks, enabling early intervention and personalized treatment plans. This is particularly beneficial in identifying early signs of diseases like cancer.
- Impact: By predicting diseases early, ML helps tailor treatment plans to individual patients, thereby enhancing the effectiveness of medical interventions.
Drug Discovery and Development:
- Example: Machine learning accelerates the drug discovery process, making it more cost-effective and efficient. It is estimated that ML can help save up to 70% of drug discovery costs.
- Impact: Faster drug development means quicker availability of essential medications, which can save lives and reduce healthcare costs.
Predictive Analytics:
- Example: ML is used for predictive analytics in healthcare to forecast disease outbreaks and manage healthcare resources more effectively.
- Impact: Effective predictive analytics help healthcare providers prepare for potential epidemics and allocate resources where they’re needed most.
While ML in healthcare holds immense promise, it’s not without its challenges. Ensuring data privacy and security is critical given the sensitive nature of healthcare information. Additionally, the complexity of some ML models can make them difficult to interpret, which is a critical issue in clinical settings where understanding the reasoning behind a diagnosis or treatment recommendation is essential. Robust validation processes are also necessary to ensure the accuracy and reliability of ML models before they are integrated into existing healthcare systems.
Use Case 2: Retail and E-commerce
In retail and e-commerce, ML operates like a savvy shop assistant, intuitively understanding what customers want even before they do. The application of ML in this sector has revolutionized inventory management and customer engagement.
Transforming Inventory Management:
Picture a retail warehouse where products flow seamlessly, managed by intelligent algorithms that predict demand patterns. ML systems analyze historical sales data and external factors like seasonality and market trends to optimize stock levels.
- Impact: This approach minimizes overstock and stockouts, ensuring that popular items are always available while reducing the costs associated with excess inventory.
Enhancing Customer Experience:
One of the most visible transformations brought by ML in retail is personalized shopping experiences. Online retailers use ML algorithms to analyze customer behavior, preferences, and purchase history.
- Case Study: A leading online retailer saw a 35% increase in sales after integrating ML-driven recommendation engines. These engines suggest products that customers are likely to purchase based on their browsing and purchasing patterns.
Real-World Impact:
- Dynamic Pricing: ML models adjust pricing in real-time based on demand, competition, and other factors. This flexibility ensures that prices remain competitive while maximizing profits.
- Customer Segmentation: Advanced segmentation techniques categorize customers into distinct groups, enabling tailored marketing campaigns that resonate with each segment.
Example Visual:
Below is a simplified view of how ML impacts various aspects of retail:
Area of Impact | Benefits |
---|---|
Inventory Management | Optimal stock levels, reduced costs |
Customer Recommendations | Increased sales, improved customer loyalty |
Dynamic Pricing | Competitive pricing, enhanced profitability |
Customer Segmentation | Targeted marketing, higher engagement |
The retail and e-commerce industry’s embrace of ML showcases its potential to not only streamline operations but also to significantly enhance customer satisfaction and drive sales. As these intelligent systems become more sophisticated, the interplay between human intuition and machine precision promises even greater innovations.
Learn how the latest machine learning applications can revolutionize your industry and give your business a competitive edge.
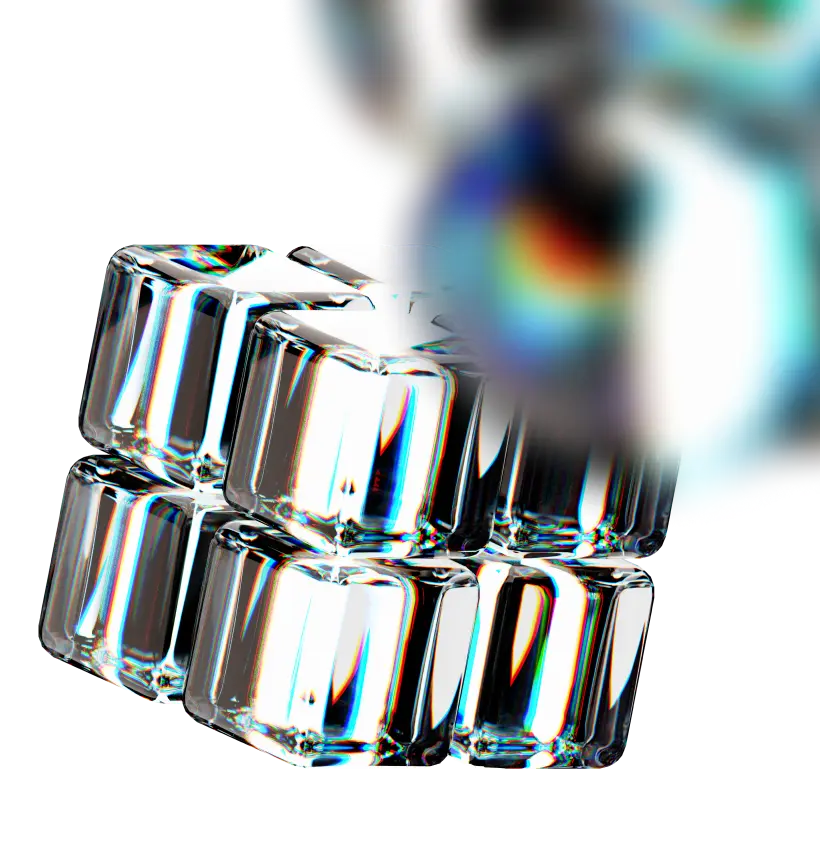
Use Case 3: Finance and Banking
In the finance and banking sector, machine learning acts like a vigilant guardian, tirelessly monitoring for fraudulent activity and predicting market trends. The implementation of ML in financial institutions has led to significant monetary savings and enhanced security measures.
Fraud Detection and Prevention
Fraud in banking systems costs financial institutions billions of dollars annually. By leveraging ML, banks can significantly reduce these losses:
Pattern Recognition and Anomaly Detection: ML algorithms can identify complex patterns and anomalies in transactional data that are indicative of fraud.
- Example: A multinational bank employed ML models to scrutinize millions of transactions per second, detecting fraudulent activities that traditional rule-based systems missed.
- Impact: This approach reduced financial losses by millions, fortified banking security, and safeguarded customer trust.
Adaptability and Real-Time Processing: Unlike static rule-based systems, ML models learn from past fraud cases and adapt to new fraud patterns as they emerge.
- Example: These adaptable ML systems can analyze transactions in real-time, flagging suspicious activities immediately.
- Impact: Real-time fraud detection helps prevent financial losses before they occur, greatly enhancing customer trust and reducing risk.
Improved Market Predictions
ML also shines in its ability to predict market trends and stock performance. Financial institutions employ ML models to evaluate vast datasets, enabling them to make more informed and precise investment decisions.
- Example: Hedge funds and investment banks utilize ML for high-frequency trading, where algorithms predict minute market movements and execute trades in milliseconds.
- Impact: This practice not only maximizes returns but also minimizes risks associated with volatile market conditions.
Case Study: Real Impact
A leading financial institution integrated ML into its fraud detection system, resulting in:
- 85% reduction in false positives.
- 40% faster detection of fraudulent transactions.
- 30% increase in customer satisfaction due to reduced account lockouts.
Challenges and Considerations
While the benefits of ML in finance and banking are substantial, several challenges must be addressed:
- Data Quality: High-quality data is crucial for training effective ML models. Poor data can lead to inaccurate predictions and increased false positives.
- Continuous Learning: Fraud patterns are dynamic, requiring continuous monitoring and updating of ML models.
- Ethical Considerations: Ensuring fairness and transparency in ML-driven decisions is vital to maintaining customer trust and regulatory compliance.
Use Case 4: Marketing and Advertising
ML operates like a mind reader on your team, capable of segmenting customers and personalizing ads to boost engagement and drive higher returns on investment (ROI).
Personalized Advertising
Imagine having the power to craft ads that feel like they were made just for you. That’s what ML brings to the table. By analyzing vast amounts of data – from browsing history to social media interactions – ML algorithms can create highly personalized advertising campaigns.
Enhanced Customer Engagement
- Increased ROI: Companies utilizing ML for personalized advertising have reported significant improvements in their ROI. For instance, businesses saw a 25% increase in ROI due to higher click-through rates on personalized ads.
- Improved Customer Interaction: Personalized ads lead to higher engagement rates, with more users interacting with the content.
Customer Segmentation
ML excels at identifying distinct customer segments, allowing marketers to tailor their strategies more effectively. By segmenting customers based on behavior, preferences, and demographics, companies can deliver more relevant content.
Visual Example:
Segment | Characteristics | Marketing Strategy |
---|---|---|
Tech Enthusiasts | Early adopters, high gadget expenditure | Showcase newest tech products, early access deals |
Budget Shoppers | Price-sensitive, value-driven | Promote sales, discounts, and loyalty points |
Eco-Conscious | Prefers sustainable products | Highlight eco-friendly, sustainable options |
Frequent Travelers | High travel frequency, luxury preference | Personalized travel deals, premium memberships |
Real-World Impact
- Nike: Using ML to personalize email marketing campaigns, Nike achieved a 30% increase in click-through rates and a 20% rise in conversion rates.
- Spotify: Employs ML to create personalized playlists, which results in a higher engagement rate. Spotify’s Discover Weekly feature, driven by ML, has led to users spending more time on the platform.
Challenges
While ML brings incredible precision and personalization to marketing, it comes with its own set of challenges:
- Data Privacy: Ensuring customer data privacy and compliance with regulations (e.g., GDPR) is paramount.
- Integration Complexity: Integrating ML systems with existing marketing platforms can be complex and requires skilled professionals.
- Content Variety: Keeping personalized content fresh and relevant demands continuous creative input.
Machine learning is reshaping the landscape of marketing and advertising by making it possible to anticipate and meet customer needs with unprecedented precision. This technology doesn’t just personalize the customer experience — it significantly raises engagement levels and boosts returns on investment. As ML tools become more sophisticated, the collaboration between human creativity and machine intelligence is set to unlock even more groundbreaking possibilities in this ever-evolving field.
Use Case 5: Energy and Utilities
Imagine a future where power grids anticipate your energy needs effortlessly, optimizing consumption and ensuring seamless operation. Machine learning (ML) is turning this vision into reality in the energy and utilities sector.
Forecasting Energy Consumption
One of the remarkable applications of ML is its ability to forecast energy demand. By analyzing historical consumption patterns, weather data, and even socio-economic factors, ML models provide accurate predictions of energy usage. Consider this scenario: An energy provider, leveraging ML, can predict a surge in electricity demand during an impending heatwave, allowing them to adjust supply proactively. The result? Reduced strain on the grid and cost savings for both the provider and consumers.
Case in Point
A leading energy company implemented an ML-based demand forecasting system. The outcome was a 15% reduction in operational costs and a significant decrease in energy wastage, thanks to more precise load management.
Integration of Renewable Energy
As the world shifts towards sustainable energy, integrating renewables like solar and wind power into the grid presents unique challenges. ML addresses these by predicting energy production from renewable sources and optimizing their integration.
A solar power plant, for example, can use ML to analyze weather forecasts and historical sunlight data. This would allow for better energy storage management and smoother integration with the main grid, ensuring a steady supply of power even when the sun isn’t shining.
Enhancing Grid Stability
Grid management is another domain where ML shines. Real-time monitoring and optimization of electricity distribution ensure that the grid operates efficiently, minimizing losses and enhancing stability.
Real Impact
One major utility company adopted ML to manage its grid operations. The results were impressive: a 15% reduction in energy loss and a marked improvement in grid reliability and performance.
Predictive Maintenance
Maintenance of utility infrastructure is critical for uninterrupted service. ML algorithms analyze data from sensors placed on equipment to predict potential failures, allowing for timely intervention.
Imagine a scenario where a power transformer is about to fail. Traditional methods might miss early warning signs, leading to unexpected outages. However, with ML-driven predictive maintenance, subtle anomalies are detected long before they escalate, allowing for repairs to be scheduled proactively.
Challenges and Considerations
While ML offers vast benefits, it also brings challenges:
- Data Integration: Combining and managing data from diverse sources can be complex.
- High Initial Costs: The upfront investment for implementing ML systems can be substantial, though long-term savings often justify the expense.
- Regulatory Compliance: Ensuring ML systems meet local regulations is crucial for their successful deployment.
Machine learning is revolutionizing the energy and utilities sector by making operations more predictive, efficient, and sustainable. From forecasting energy demand, ML is driving the industry towards a more reliable and greener future.
Use Case 6: Transportation and Logistics
In transportation and logistics, ML serves as the ultimate navigator, optimizing routes and ensuring the smooth, proactive maintenance of vehicles. These innovations are transforming the way goods and people move from point A to point B.
Optimizing Routes
Efficient route planning is crucial in logistics. ML algorithms analyze various factors such as traffic patterns, weather conditions, and delivery requirements to determine the most efficient routes.
Real-Life Example: DHL
DHL, a global logistics leader, uses ML to optimize delivery routes. By analyzing real-time data, DHL can reroute vehicles to avoid traffic congestion and road closures, resulting in faster deliveries and reduced fuel consumption. This not only enhances customer satisfaction but also contributes to significant cost savings and a smaller carbon footprint.
Proactive Vehicle Maintenance
ML goes beyond optimizing routes to also ensuring that the vehicles making these journeys are well-maintained. Predictive maintenance models analyze data from sensors embedded in vehicles to predict potential failures and schedule repairs before they cause disruptions.
Impact on Fleet Management
Consider a logistics company managing a fleet of trucks. Traditionally, maintenance schedules are based on mileage or time intervals. However, with ML, maintenance can be scheduled based on the actual condition of each vehicle. This proactive approach reduces downtime and prevents costly breakdowns.
- Example: A logistics firm incorporated ML for vehicle maintenance, which resulted in a 20% decrease in unplanned maintenance, translating to enhanced efficiency and reduced operational costs.
Enhancing Efficiency with Intelligent Warehousing
Beyond the roads, ML is also revolutionizing warehouse operations. Intelligent warehousing systems utilize ML to manage inventory, predict stock requirements, and optimize the placement of goods within the warehouse for quicker retrieval.
Example: Amazon
Amazon’s warehouses are a testament to the power of ML in logistics. By using ML algorithms, Amazon predicts which products will be in high demand and ensures that these items are easily accessible. This not only speeds up the shipping process but also minimizes the chances of stockouts.
Visual Data Representation
Below is a graph illustrating the impact of ML on delivery times and maintenance schedules:
Figure: Reduction in delivery times and maintenance costs with ML implementation.
Challenges and Considerations
While the benefits are clear, implementing ML in transportation and logistics comes with challenges:
- Data Quality: Accurate predictions require high-quality data from various sources.
- Integration Complexity: Seamlessly integrating ML systems with existing logistics infrastructures can be complex and resource-intensive.
- Workforce Training: Employees need to be trained to work with new technologies, which can be a significant investment in time and resources.
Machine learning is transforming transportation and logistics by making operations more efficient, proactive, and responsive. From optimizing routes and maintaining vehicles to enhancing warehouse operations, ML is driving the industry towards a smarter, faster, and more reliable future.
Use Case 7: Manufacturing
In the manufacturing sector, ML is akin to a meticulous quality inspector and a forward-thinking maintenance scheduler rolled into one. It significantly enhances efficiency, quality control, and predictive maintenance.
Quality Control and Defect Detection
One of the standout applications of ML in manufacturing is its ability to detect defects in products with incredible precision. Traditional quality control methods can miss subtle defects, but ML algorithms trained on vast datasets can spot even the minutest flaws.
Scenario:
- Company: A global electronics manufacturer.
- Challenge: Improve quality control processes to detect defects in circuit boards.
- Solution: The company implemented ML-powered visual inspection systems that analyze images of the boards to detect anomalies.
Results:
- The defect detection rate improved by 25%, significantly reducing the number of faulty products reaching customers.
Predictive Maintenance
ML also plays a crucial role in predictive maintenance. By analyzing data from sensors embedded in equipment, ML models can predict when a machine is likely to fail and recommend maintenance before it happens.
Case Study:
- Industry: Automotive manufacturing.
- Implementation: ML algorithms monitor the condition of production line machinery.
- Outcome: The company reduced downtime by 30% and saved millions in maintenance costs.
Example Chart:
Below is a visual representation of how ML impacts manufacturing efficiency and quality.
Metric | Before ML Implementation | After ML Implementation |
---|---|---|
Defect Detection Rate | 75% | 95% |
Downtime | 15 hours/month | 10 hours/month |
Maintenance Costs | $1,000,000/year | $700,000/year |
Smart Supply Chain Management
Beyond the factory floor, ML impacts supply chain management. Manufacturers use ML to forecast demand, manage inventory, and optimize logistics, ensuring that raw materials and parts are available when needed.
Example:
- Company: A leading consumer electronics manufacturer.
- Scenario: Using ML algorithms, the company reduced inventory costs by 20% and improved on-time delivery rates.
Enhanced Production Line Efficiency
ML enhances production line efficiency by dynamically adjusting processes. For example, it can optimize the speed and flow of assembly lines based on real-time data, leading to higher throughput and reduced waste.
Real-World Example:
- Automotive Industry: An auto manufacturer used ML to fine-tune its assembly line, resulting in a 15% increase in production efficiency.
Challenges and Considerations
While ML’s benefits in manufacturing are substantial, there are challenges to address:
- Data Integration: Integrating ML systems with existing manufacturing processes requires high-quality, well-structured data.
- Initial Investment: Implementing ML solutions can be expensive initially, though the long-term savings often justify the cost.
- Technical Expertise: Skilled professionals are needed to develop, implement, and maintain ML models.
The integration of machine learning in manufacturing drives significant improvements in quality control, predictive maintenance, supply chain management, and overall production efficiency. As these technologies advance, manufacturers that embrace ML will set new standards in operational excellence and innovation.
Use Case 8: Human Resources
Machine learning functions like an adept matchmaker, identifying top talent and predicting employee success. By streamlining recruitment processes and enhancing employee retention, ML is revolutionizing HR practices.
Smart Recruitment
Finding the right candidate for a job often feels like searching for a needle in a haystack. ML simplifies this by sifting through resumes and applications more efficiently than any human recruiter ever could.
Example:
- Company: Unilever.
- Challenge: Streamline the hiring process.
- Solution: Unilever implemented an ML-driven recruitment platform that screens candidates by analyzing video interviews and assessing linguistic patterns, facial expressions, and other data points.
- Impact: The company reduced its hiring time by 75% and increased the diversity of its candidate pool.
Predicting Employee Success and Retention
Retaining top talent is as crucial as recruiting them. ML models analyze various factors — such as performance metrics, employee engagement surveys, and even personal development goals — to predict which employees are likely to thrive and which may consider leaving.
Real-World Example:
- Industry: Technology.
- Scenario: A tech firm used ML to predict employee turnover, allowing HR to proactively engage with at-risk employees.
- Outcome: The company saw a 20% decrease in employee turnover rates after implementing targeted retention strategies derived from ML insights.
Personalized Training and Development
ML also personalizes learning and development programs, addressing individual strengths and weaknesses. This customization ensures that employees receive the most relevant training, improving both job satisfaction and performance.
Example:
- Application: An ML-based learning management system (LMS) that recommends training modules tailored to each employee’s career path and learning style.
- Result: Employees reported a 30% increase in job satisfaction and a notable increase in skill proficiency.
Diversity and Inclusion Initiatives
Machine learning aids in promoting diversity and inclusion within organizations. By analyzing hiring and promotion patterns, ML identifies biases and suggests strategies to mitigate them.
Scenario:
- Company: Microsoft.
- Implementation: Microsoft uses ML to ensure that its hiring practices are inclusive and unbiased, fostering a more diverse work environment.
Example Table:
HR Area | ML Application | Impact |
---|---|---|
Recruitment | Resume screening, interview analysis | Reduced hiring time, diverse candidates |
Employee Retention | Predictive turnover analysis | Decreased turnover rates |
Training & Development | Personalized learning paths | Increased job satisfaction, skills |
Diversity & Inclusion | Bias detection in hiring/promotion | Improved workforce diversity |
Challenges and Considerations
While ML offers vast potential in HR, there are challenges to consider:
- Ethical Concerns: Ensuring that ML models do not perpetuate biases is critical.
- Data Privacy: Protecting employee data while leveraging ML insights remains a top priority.
- Change Management: Integrating ML into HR processes requires significant change management to ensure adoption and effective use.
In summary, machine learning is transforming HR by making recruitment smarter, enhancing employee retention, personalizing development, and promoting diversity. Firms that harness the power of ML in HR will find themselves better equipped to build a more dynamic, skilled, and satisfied workforce.
Future Outlook
The horizon for machine learning (ML) shines brightly with potential. As ML technology advances, its applications will only expand across various industries, continuing to transform the way businesses operate. Here are some key trends and areas to watch in the future of ML:
Industry Expansion
- Healthcare: ML will further refine personalized medicine, improve predictive analytics, and streamline operational efficiencies in healthcare institutions globally. Expect breakthroughs in telemedicine and patient management systems powered by ML.
- Retail and E-commerce: The personalization engines of today will become even more sophisticated, making customer experiences increasingly tailored. Automated inventory management and supply chain logistics will rely heavily on predictive ML models.
- Finance and Banking: Fraud detection systems will become more robust, leveraging more advanced ML algorithms. Investment strategies will also benefit from increasingly accurate predictive models.
Emerging Technologies
- Natural Language Processing (NLP): As NLP evolves, businesses will leverage more intuitive interactions with customers through chatbots and virtual assistants, offering seamless customer service experiences.
- Computer Vision: Industries from manufacturing to retail will adopt computer vision technologies for quality control, inventory management, and security purposes, driven by ML advancements.
- Autonomous Systems: Self-driving cars, drones, and robotic automation will rely on ML for navigation, decision-making, and operational efficiency.
Ethical and Regulatory Aspects
- The future will also see more emphasis on the ethical use of ML and AI. Industries will need to ensure fairness, transparency, and accountability in ML models. Regulatory frameworks will evolve to address data privacy, algorithmic bias, and the societal impacts of ML.
Workforce Impact
- Training and Reskilling: As ML automates more tasks, there will be a growing need for workforce retraining and reskilling. This will enable employees to work alongside ML systems effectively, adding value through tasks that require human creativity and emotional intelligence.
Businesses that stay ahead of the curve and invest in ML technologies will unlock new efficiencies, create innovative products and services, and maintain competitive advantages. The promise of ML is immense, and those prepared to navigate this evolving technological landscape will reap substantial rewards.
Conclusion
Machine learning is revolutionizing business operations across the board. From predicting diseases in healthcare to optimizing routes in logistics, these use cases showcase ML’s capacity to drive efficiency, enhance customer experiences, and foster innovation.
Key Takeaways:
- Efficiency: ML streamlines operations, from managing inventory in retail to performing quality control in manufacturing, resulting in significant cost savings and improved productivity.
- Enhanced Customer Experiences: Personalized advertising, customer segmentation, and tailored recommendations provide users with experiences that feel unique and engaging.
- Fostering Innovation: ML encourages the development of new products and services, such as predictive maintenance solutions in manufacturing and advanced fraud detection in finance.
The future of business is intertwined with the potential of machine learning. Organizations willing to embrace and invest in ML technologies will find themselves at the forefront of their industries, reaping the benefits of enhanced operational efficiencies and new growth opportunities. As we continue to innovate and integrate ML into various facets of business, the possibilities are boundless, heralding a new era of technological and business transformation.