AI in Demand Forecasting: How Predictive Analytics Optimizes Business Operations
In today’s rapidly evolving marketplace, businesses must accurately predict customer demand to maintain a competitive edge. Relying on traditional forecasting methods often results in inefficiencies such as surplus inventory, unexpected shortages, and financial losses. AI-powered demand forecasting tools are revolutionizing business forecasting by enabling companies to make intelligent, data-driven decisions that enhance inventory management, streamline operations, and cut unnecessary costs.
By leveraging machine learning and AI tools, businesses can analyze historical sales data, anticipate market fluctuations, adapt to seasonal trends, and swiftly respond to unforeseen disruptions. Instead of reacting to changes after they occur, AI-driven forecasting empowers organizations to take a proactive approach, fine-tuning their strategies in real time to improve efficiency, maximize profitability, and meet future customer demand with greater precision.
The Role of AI in Demand Forecasting
AI-powered demand forecasting utilizes predictive modeling and sophisticated algorithms to assess past sales trends, analyze consumer purchasing habits, track market shifts, and account for external influences such as economic changes and seasonal patterns. This advanced approach significantly enhances prediction accuracy, enabling businesses to refine their strategies and maintain optimal efficiency.
Optimizing Inventory Management
AI demand forecasting tools help businesses predict demand fluctuations with high precision, reducing the risk of surplus inventory or unexpected stock shortages. This ensures that the right products are always available at the right time, improving customer satisfaction while enhancing cash flow and minimizing unnecessary storage costs.
Boosting Operational Efficiency
Predictive analytics helps companies allocate resources more effectively by aligning staffing, logistics, and production schedules with anticipated demand. By reducing excess labor costs and optimizing workflows, businesses can operate more efficiently and minimize waste, resulting in leaner, more cost-effective operations.
Refining Pricing Strategies
AI-based demand forecasting enables companies to implement dynamic pricing models that adjust in real time based on demand trends, competitor pricing, and market conditions. This data-driven approach ensures that businesses can maximize profitability while maintaining competitive pricing that appeals to consumers.
Companies like Uber and airlines utilize AI-driven forecasting models to adjust fares based on demand fluctuations in real time. For example, during peak hours or high-demand travel seasons, AI algorithms analyze historical sales data, booking trends, competitor prices, and other factors to dynamically adjust pricing. This ensures maximum revenue generation while optimizing future customer demand. Similarly, e-commerce platforms employ AI-powered predictive modeling to modify pricing based on competitor actions and demand trends, helping businesses remain competitive and profitable.
Enhancing Supply Chain Coordination
The integration of AI into demand forecasting improves supply chain management by predicting demand shifts and optimizing inventory distribution. Leading logistics companies use AI-powered forecasting tools to analyze vast datasets, anticipate market demand, and optimize resource allocation. This minimizes operational bottlenecks and enhances delivery efficiency, ensuring seamless logistics operations.
As businesses continue to harness the power of AI, integrating predictive analytics into strategic planning will be essential for achieving accurate demand forecasts, improving financial forecasting, and maintaining a competitive edge.
Case Study: FedEx and AI-Driven Demand Forecasting
FedEx leverages AI-powered predictive analytics to optimize its logistics network. By analyzing vast datasets, including historical shipping patterns, weather conditions, and economic indicators, FedEx can anticipate surges in demand and allocate resources more effectively. For example, during peak seasons like the holiday rush, AI-driven demand forecasting enables FedEx to proactively adjust staffing, reroute shipments, and optimize warehouse operations, ensuring timely deliveries and reducing operational costs. (Source, Source)
Similarly, DHL integrates AI into its demand forecasting models to enhance supply chain efficiency. Using machine learning algorithms, DHL predicts fluctuations in package volumes, allowing for dynamic route planning and load balancing. This AI-driven approach not only minimizes delays but also improves sustainability by reducing fuel consumption and optimizing vehicle usage. (Source, Source)
These implementations highlight how logistics giants are using AI to streamline supply chain coordination and improve overall efficiency.
Improving Customer Satisfaction
Accurate demand forecasting prevents frustrating stockouts and overstock situations, ensuring that customers always find the products they need when they need them. Additionally, AI-powered insights help businesses personalize marketing efforts, offering targeted promotions and recommendations that enhance customer engagement and loyalty.
Our technical team specializes in data integration, AI model selection, and change management to ensure a smooth transition to AI-powered forecasting for your business
AI vs. Traditional Forecasting Methods
Traditional demand forecasting processes typically rely on historical sales trends and manual statistical analysis. While these conventional forecasting techniques provide a foundational understanding of market demand, they often struggle to account for rapidly evolving consumer behavior, external disruptions, and real-time market dynamics. Demand forecasting using AI and machine learning leverages automation, predictive analytics, and big data to offer accurate forecasting, greater efficiency, and scalability.
Higher Accuracy Through Continuous Learning
Unlike traditional models that rely on static data, AI continuously processes and learns from new information. By refining its predictions based on real-time market conditions, AI improves the accuracy of demand forecasts, enabling businesses to accurately predict demand and adjust strategies accordingly.
Faster Decision-Making with Real-Time Processing
AI enables businesses to analyze vast amounts of data in real time, allowing for rapid adjustments to inventory levels, pricing, and supply chain logistics. Traditional forecasting relies on periodic updates, making it less responsive to shifts in demand and unexpected disruptions.
Uncovering Hidden Trends and Patterns
AI excels at identifying intricate patterns in customer behavior, purchasing habits, and market fluctuations. By forecasting by analyzing diverse datasets, businesses can anticipate future trends and proactively adjust their business strategies to stay competitive.
Eliminating Human Bias for Objective Insights
Traditional demand forecasting involves human judgment, which can introduce bias. AI provides objective, data-driven insights, ensuring more precise demand forecasts and reducing costly forecasting errors.
Scalability for Complex Business Needs
AI-powered models seamlessly scale with business growth and market expansion, making them ideal for enterprises with extensive product portfolios, multiple sales channels, or global supply chains. Forecasting relies on AI’s ability to process massive datasets efficiently, whereas traditional methods often struggle with complexity.
Real-World Applications of AI in Demand Forecasting
AI-driven demand forecasting is revolutionizing multiple industries by providing data-driven insights, reducing inefficiencies, and improving decision-making. Businesses across various sectors are leveraging AI to enhance operational performance and better meet customer demand.
Retail & E-Commerce
Retailers use AI to predict demand patterns, ensuring optimal stock levels and minimizing overstock or shortages. AI-powered recommendation engines personalize shopping experiences by analyzing customer purchase history and preferences, increasing conversion rates and customer retention. Additionally, AI aids in regional inventory distribution, helping businesses allocate products efficiently across different store locations based on local demand trends.
Example: Walmart’s AI-Powered Inventory Management
Walmart utilizes artificial intelligence to enhance its inventory management system, ensuring products are available when customers need them. By analyzing historical data and employing predictive analytics, Walmart strategically positions items across distribution centers and stores, optimizing the shopping experience. (source)
Manufacturing
AI helps manufacturers anticipate material needs, preventing shortages and excess inventory. By analyzing production cycles, supply chain logistics, and historical data, AI reduces bottlenecks and enhances workflow efficiency. Additionally, predictive maintenance capabilities detect equipment failures before they occur, reducing downtime and optimizing production schedules.
Example: Siemens’ AI-Driven Production Planning
Siemens integrates AI technologies into its production planning to optimize resource allocation, reduce downtime, and improve overall efficiency. By leveraging AI, Siemens enhances manufacturing processes, leading to increased productivity and cost savings. (source)
Logistics & Supply Chain
AI-driven forecasting empowers logistics companies to streamline warehouse management, optimize transportation routes, and improve delivery efficiency. By analyzing real-time demand data, AI helps reroute shipments, balance inventory across distribution centers, and reduce transportation costs. This proactive approach minimizes disruptions and ensures smooth supply chain operations.
Healthcare
Hospitals and pharmaceutical companies leverage AI to predict patient admission rates, ensuring proper staffing and resource allocation. AI-driven demand forecasting also helps maintain optimal stock levels of medical supplies and medications, reducing waste and preventing shortages. This improves patient care while reducing operational inefficiencies.
Financial Services
Banks and financial institutions use AI to analyze economic trends, customer spending habits, and credit risks. By predicting demand for loans, investment products, and financial services, AI enhances risk management and decision-making. Financial institutions can also adjust interest rates and lending policies dynamically based on AI-driven market insights.
Hospitality & Travel
Hotels and airlines utilize AI to forecast occupancy rates, optimize pricing strategies, and plan staffing levels based on seasonal and real-time demand fluctuations. AI-driven dynamic pricing models help businesses adjust rates based on customer demand, competitor pricing, and external factors like holidays or events, maximizing profitability while maintaining customer satisfaction.
Implementing AI for Demand Forecasting in Your Business
Integrating AI-driven demand forecasting into business operations requires a structured approach to ensure accuracy, efficiency, and scalability. By following key steps, companies can harness AI’s full potential to optimize inventory, improve supply chain management, and enhance decision-making.
Step 1: Gathering and Integrating Data
Successful AI forecasting begins with collecting relevant data from diverse sources, such as past sales records, industry trends, consumer purchasing habits, and external factors like economic indicators and seasonality. Consolidating this information into a unified platform ensures a holistic view of demand patterns and enables more precise predictions. Seamless integration across databases, ERP systems, and analytics tools is crucial for effective analysis.
Get a personalized roadmap for implementing AI-driven forecasting solutions tailored to your industry needs from our data science experts
Step 2: Choosing the Appropriate AI Model
Selecting the right AI model depends on business needs and industry dynamics. Various AI approaches include:
Time Series Analysis: Uses historical trends to predict future demand, ideal for businesses with stable, cyclical sales patterns.
Machine Learning Techniques: Recognizes hidden correlations and anomalies within datasets, refining forecasts by identifying emerging trends.
Deep Learning Frameworks: Processes vast amounts of data to detect intricate patterns, particularly beneficial for industries experiencing frequent demand shifts.
Step 3: Model Training and Validation
After choosing an AI model, it must be trained with historical and real-time data to enhance accuracy. Continuous testing and refinement ensure that the model aligns with actual market behavior. Performance validation involves comparing predictions against real outcomes, adjusting parameters, and fine-tuning the model to minimize errors.
Step 4: Integrating AI into Business Processes
For AI forecasting to drive tangible benefits, it must be embedded into critical operations like inventory control, logistics coordination, and dynamic pricing strategies. Ensuring that employees understand how to interpret and apply AI-driven insights is essential for maximizing its impact. Businesses should also develop workflows that allow AI outputs to inform decision-making in real time.
Step 5: Monitoring, Updating, and Optimizing
AI-driven models require continuous oversight to maintain their effectiveness in changing market environments. Regular updates, recalibration, and the incorporation of new data sources enhance long-term accuracy. Establishing feedback mechanisms allows businesses to refine their AI strategies over time, ensuring sustained improvements in forecasting precision and operational efficiency.
Challenges of AI in Demand Forecasting
While AI-driven demand forecasting enhances accuracy and efficiency, its implementation presents several hurdles that businesses must address to maximize its effectiveness.
1. Data Quality and Accessibility
AI models thrive on accurate, well-structured data. However, poor data quality — such as incomplete, outdated, or inconsistent records — can undermine forecasting reliability. To mitigate this risk, businesses must invest in rigorous data validation, cleansing, and integration strategies to ensure that AI systems operate with reliable inputs.
2. High Implementation Costs
Deploying AI-powered forecasting requires financial investment in advanced software, infrastructure, and skilled personnel. While the upfront costs can be significant, the long-term gains — such as reduced waste, optimized inventory, and improved decision-making — often justify the initial expenditure. Companies should weigh these benefits when evaluating AI adoption.
3. Resistance to Change
Employees may be hesitant to embrace AI-driven insights, particularly if they fear automation will replace traditional decision-making roles. Effective change management involves providing training, demonstrating AI’s value, and fostering a culture that encourages human-AI collaboration rather than replacement.
4. Ethical and Bias Considerations
AI algorithms must be designed and monitored to ensure fairness and prevent biased decision-making. Bias in historical data can lead to skewed predictions, affecting everything from pricing strategies to inventory planning. A notable example is Amazon’s AI-powered hiring tool, which was scrapped after it was found to favor male candidates over female ones due to biases in historical hiring data. Similarly, biased demand forecasts can result in overstocking in certain markets while underserving others, creating inefficiencies and revenue loss. Regular audits, transparent methodologies, and responsible AI governance are essential to maintaining ethical forecasting practices.
Future of AI in Demand Forecasting
AI-driven demand forecasting is rapidly evolving, offering businesses increasingly sophisticated tools to navigate market uncertainties. Future advancements in AI will enhance forecasting accuracy, streamline operations, and boost overall business resilience. With continuous improvements in deep learning, automation, and real-time analytics, organizations will be better equipped to anticipate demand fluctuations and optimize their strategies.
Emerging Trends
Automated AI Forecasting: AI systems will become more self-sufficient, reducing the need for manual oversight. With improved learning algorithms, AI will automatically refine predictions, adapt to changing patterns, and enhance forecasting precision without constant human intervention.
Integration with IoT: The rise of smart sensors and Internet of Things (IoT) devices will revolutionize demand forecasting. Real-time data from connected devices — such as smart shelves, RFID tags, and weather sensors — will provide businesses with granular insights, enabling more accurate and timely demand predictions.
AI-Driven Supply Chains: AI will transform supply chain management by optimizing coordination between suppliers, manufacturers, and distributors. Predictive analytics will enhance demand planning, reduce bottlenecks, and minimize delays, ensuring a more responsive and efficient supply chain.
Explainable AI: As AI becomes a crucial part of business decision-making, companies will demand greater transparency in AI-driven forecasts. Explainable AI will allow businesses to understand how predictions are made, fostering trust, regulatory compliance, and improved decision-making.
Our experienced development team can help you design and implement custom AI forecasting solutions that enhance inventory management, optimize supply chains, and drive business growth.
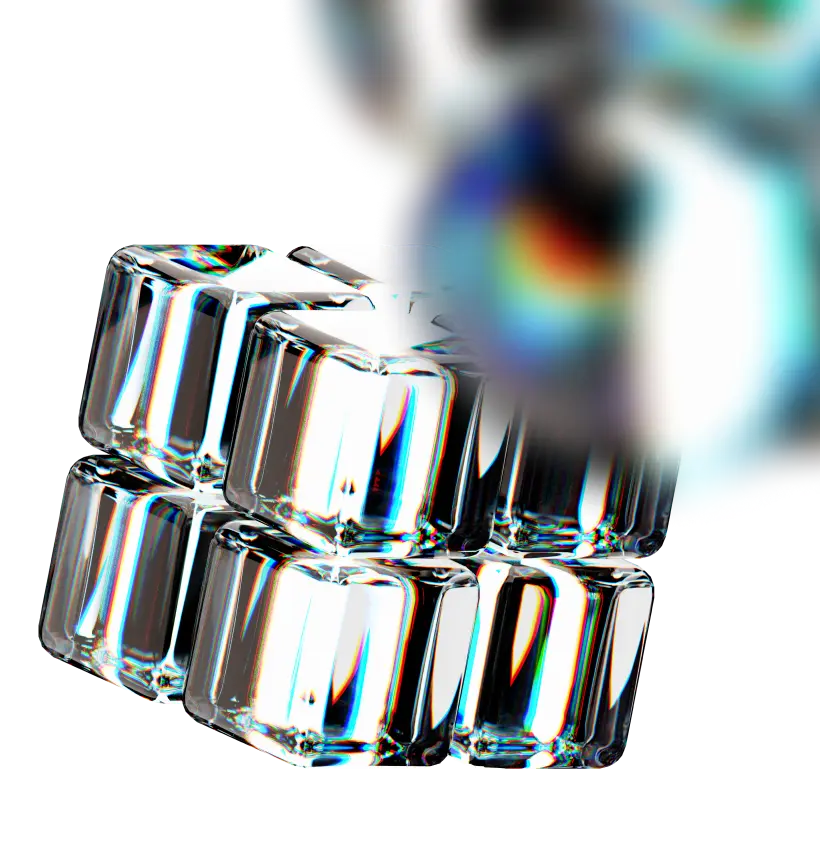
Embracing AI for Smarter Demand Forecasting
AI-driven demand forecasting is revolutionizing the way businesses anticipate market trends, optimize inventory, and allocate resources. By leveraging advanced predictive analytics, companies can make smarter, data-driven decisions that enhance efficiency, reduce costs, and boost profitability. With AI’s ability to adapt to real-time changes and uncover hidden patterns, businesses can stay agile in an increasingly dynamic marketplace.
Attract Group specializes in designing AI-powered forecasting solutions tailored to your business needs. Whether you want to improve inventory management, streamline supply chains, or enhance operational efficiency, our expertise can help you implement cutting-edge AI models. Contact us today to discover how AI can transform your business and give you a competitive edge.