Applications of Machine Learning in Healthcare: Benefits & Case Studies
Have you ever wondered how a machine can predict if someone has a disease just by analyzing their medical data? Welcome to the world of machine learning in healthcare, a revolutionary approach that’s transforming patient care and the healthcare industry as a whole. With the burgeoning field of artificial intelligence (AI), machine learning (ML) stands at the forefront, offering unprecedented capabilities to improve healthcare delivery, enhance patient outcomes, and streamline operations.
Machine learning, a subset of artificial intelligence, empowers systems to learn from data, identify patterns, and make decisions with minimal human intervention. In the healthcare sector, the impact of these technologies is both profound and far-reaching. From diagnosing diseases with pinpoint accuracy to devising personalized treatment plans, ML in healthcare embodies the future of medicine.
The purpose of this article is to explore the applications of machine learning in the healthcare industry, delve into the benefits it offers, and highlight real-world case studies showcasing its transformative power. We’ll take you through various aspects of machine learning applications in healthcare, uncovering how these innovative technologies are reshaping the ways healthcare organizations operate and care for their patients.
Understanding Machine Learning
So, what exactly is this magical force called machine learning that’s stirring waves in the healthcare world? At its core, machine learning is a branch of artificial intelligence where computer systems learn from data and improve their predictions or behaviors over time. Imagine teaching a child to ride a bike: after a few wobbly attempts and a couple of falls, the child learns to maintain balance, steer, and pedal more efficiently. Similarly, machine learning algorithms improve their performance as they process more data.
Types of Machine Learning
Not all machine learning is created equal. There are three primary types of machine learning:
Supervised Learning: Think of this as a teacher-student scenario. Here, the machine learning model is trained on a labeled dataset, where the correct output is known. It learns to map inputs to outputs, much like a student learning to solve math problems with given answers. This is extensively used in diagnostics, where models can identify diseases by learning from labeled medical images.
Unsupervised Learning: Imagine a curious explorer venturing into an unknown territory without any guide. Unsupervised learning algorithms work on unlabeled data and find hidden patterns or intrinsic structures within the data. This approach is valuable in healthcare for clustering patient data to find new disease subtypes or for anomaly detection in medical records.
Reinforcement Learning: Picture training a dog using a series of rewards and penalties. Reinforcement learning involves training an agent through rewards for good actions and penalties for bad actions. This type of machine learning is making strides in optimizing treatment plans and robotic surgeries where the learning model improves by getting feedback from each action.
Key Algorithms Used in Healthcare
Healthcare machine learning doesn’t work without the heavy-lifting by robust algorithms. Here are a few heroes in the ML arsenal:
- Decision Trees: Simple yet effective, decision trees split data into branches to make predictions. They are used for clinical decision support.
- Neural Networks: Mimicking the human brain, these networks are fantastic for recognizing complex patterns, such as identifying cancerous cells in medical images.
- Support Vector Machines (SVM): Used extensively in genomics, these algorithms classify patient data by finding the optimal boundary between data points.
- K-means Clustering: A popular unsupervised learning method used to group patients based on similar traits or symptoms.
These algorithms and more are the backbone of how machine learning can help sift through vast amounts of healthcare data, making intelligent predictions and decisions that benefit both healthcare providers and patients.
Machine learning in healthcare is like having a team of super-intelligent assistants tirelessly working around the clock, analyzing every bit of information to offer the best possible outcomes. Exciting, isn’t it?
Machine Learning Benefits
Machine learning in healthcare isn’t just a shiny new toy; its benefits ripple across the entire healthcare spectrum, creating waves of positive change. The advantages are manifold, and they touch upon every aspect of healthcare, from enhancing diagnostics to optimizing treatment plans. Let’s delve into some of the most impactful benefits.1.
1. Improved Diagnostics
One of the most significant benefits of machine learning is its ability to improve diagnostic accuracy. Machine learning algorithms can analyze complex medical data, such as medical images and genetic information, with a level of precision that often surpasses human capabilities. For instance, deep learning models can examine X-rays, MRIs, and CT scans to detect anomalies, such as tumors or fractures, that might be challenging for the human eye to discern. This leads to earlier and more accurate diagnoses, which can be lifesaving in critical conditions like cancer.
2. Personalized Treatment Plans
In the realm of treatment, one-size-fits-all is increasingly becoming a thing of the past. Machine learning allows healthcare providers to devise personalized treatment plans tailored to individual patient needs. By analyzing a patient’s genetic makeup, medical history, and real-time health data, machine learning models can recommend the most effective therapies. This even extends to predicting how a patient might respond to specific treatments, thereby minimizing trial-and-error and optimizing recovery times.
3. Enhanced Patient Monitoring
Machine learning shines in continuous patient monitoring, a critical aspect of managing chronic illnesses and post-operative care. Wearable devices equipped with ML algorithms can monitor vital signs in real-time and alert healthcare professionals to any concerning changes. This proactive approach helps in early detection of potential health issues, enabling timely interventions and reducing hospital readmissions.
4. Operational Efficiency
Beyond patient care, machine learning significantly boosts operational efficiency in healthcare organizations. For example, ML algorithms can streamline administrative tasks such as patient record management, billing, and appointment scheduling. This not only frees up valuable time for healthcare professionals but also reduces operational costs. Furthermore, predictive analytics can optimize resource allocation in hospitals, ensuring that staff, equipment, and facilities are utilized most effectively.
5. Cost Reduction
Finally, the financial benefits cannot be overlooked. Implementing machine learning can lead to substantial cost savings by reducing diagnostic errors, optimizing treatment plans, and improving operational efficiencies. These savings can be reinvested in further innovations and improvements within the healthcare system, creating a virtuous cycle of advancement and improvement.
The benefits of machine learning in healthcare are profound and multifaceted, offering a glimpse into a future where technology and medicine work hand-in-hand to provide the best possible care. Whether through improved diagnostics, personalized treatments, enhanced monitoring, or operational efficiency, machine learning stands as a cornerstone of modern healthcare innovation.
Learn how our innovative machine learning applications can enhance patient care and optimize healthcare operations through real-world case studies.
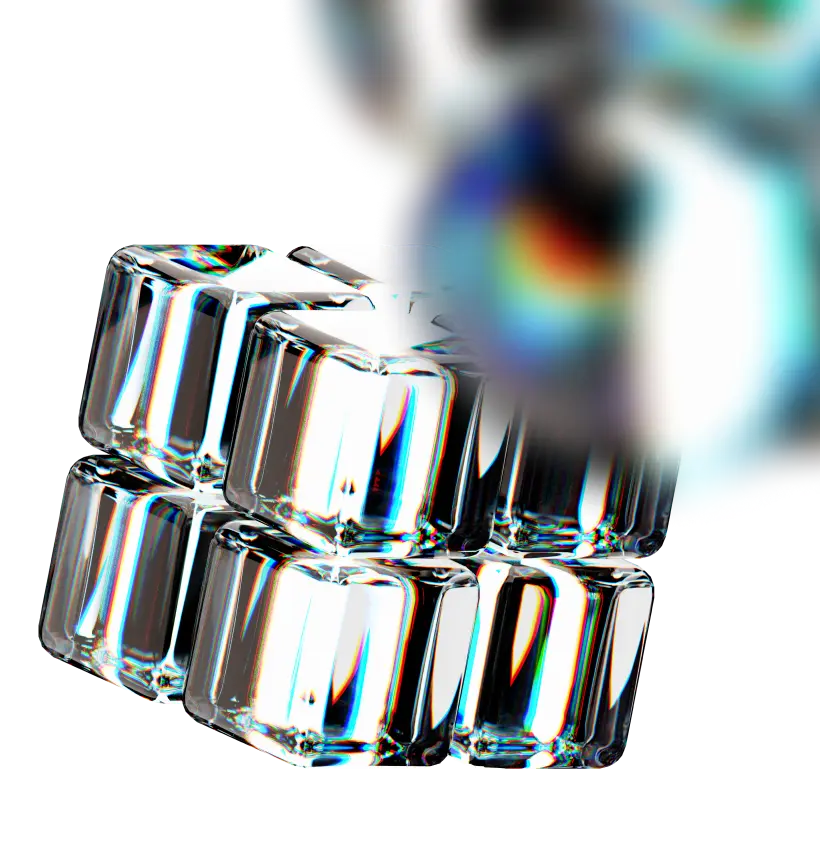
Applications of Machine Learning in Healthcare
Diagnostics
The realm of diagnostics is where machine learning truly comes into its own. Imagine a world where detecting a disease is as quick and easy as taking a snapshot. With machine learning applications in healthcare:
Image Recognition in Radiology: Advanced algorithms, particularly deep learning models, can scan through radiological images such as X-rays, MRIs, and CT scans with incredible accuracy. They can identify abnormalities like tumors, fractures, or lesions that might be missed by human eyes.
Pathology and Lab Test Analysis: Machine learning algorithms can sift through immense amounts of lab data to identify patterns that signal disease. For example, specific changes in blood tests might indicate diabetes or kidney problems, which can be caught early on.
Predictive Analytics for Disease Diagnosis: By analyzing a patient’s medical history, genetic information, and lifestyle factors, machine learning can predict the likelihood of certain diseases. This enables healthcare professionals to take preventive measures well in advance.
Treatment Plans
Creating the perfect treatment plan for a patient is akin to solving a complex puzzle, and this is where machine learning excels:
Personalized Medicine and Treatment Recommendations: Machine learning models can analyze data from thousands of medical cases to recommend treatment plans that are tailored to individual patient needs. This ensures that each patient receives the most effective therapy based on their unique characteristics.
Optimization of Treatment Protocols: Machine learning can also help in refining and optimizing existing treatment protocols, making them more effective and reducing side effects.
Monitoring Patient Response and Adjusting Treatments: Continuous monitoring allows healthcare providers to see how patients are responding to treatments. Machine learning algorithms can analyze this data to adjust therapies in real-time, ensuring better outcomes.
Patient Monitoring
The future of patient care lies in constant and precise monitoring, and machine learning is at the heart of this:
Remote Patient Monitoring and Wearable Technology: Wearable devices equipped with sensors can track patients’ vital signs in real-time. Machine learning models analyze this data to provide actionable insights, alerting healthcare professionals to potential issues before they escalate.
Predicting Patient Deterioration: Predictive analytics can identify subtle signs of patient deterioration that might be missed by intermittent checkups. This allows for timely interventions, improving patient outcomes.
Managing Chronic Diseases: Chronic diseases require ongoing management. Machine learning helps in tracking disease progression, adjusting treatments as needed, and providing personalized recommendations, making it easier for patients to manage their conditions.
Operational Efficiency
Beyond clinical applications, machine learning also plays a crucial role in enhancing operational efficiency within healthcare organizations:
Streamlining Administrative Tasks: Automating routine tasks like appointment scheduling, patient record management, and billing can save time and reduce administrative burden on healthcare professionals.
Optimizing Resource Allocation in Hospitals: Predictive models can forecast patient admissions, enabling hospitals to allocate resources more efficiently. This ensures that beds, staff, and equipment are available when needed most.
Reducing Costs and Improving Patient Outcomes: By optimizing various aspects of healthcare delivery, machine learning can reduce costs while ensuring high-quality care. This creates a win-win situation for both healthcare providers and patients.
Applications and Benefits
Genetic Data Analysis: AI and ML are used to analyze genetic data, identifying variations and correlations with specific diseases. This enables healthcare professionals to develop targeted treatments and therapies (source).
Tailored Treatment Plans: AI helps develop personalized treatment plans and drug therapies based on individual patient characteristics, medical history, and genetic profiles. This approach improves treatment outcomes and reduces side effects (source).
Patient Stratification: AI identifies patient subgroups with similar characteristics, allowing for the development of targeted interventions and treatments (source).
The applications of machine learning in healthcare are incredibly diverse and impactful, paving the way for a more efficient, effective, and patient-centric healthcare system. As we move forward, the integration of these technologies will undoubtedly transform the landscape of medicine, bringing about better health outcomes and operational efficiency.
Case Studies
Machine learning (ML) applications in personalized medicine have led to significant advancements and success stories in healthcare. These case studies not only illuminate the transformative power of machine learning in the healthcare industry but also inspire further innovations.
Success Stories in Personalized Medicine
xDECIDE by xCures
Imagine having a team of seasoned oncologists and advanced AI algorithms jointly crafting the most effective cancer treatment plan tailored just for you. Enter xDECIDE by xCures. This oncology-focused AI platform combines human expertise with AI software to offer personalized treatment options for cancer patients. Using natural language processing (NLP) and machine learning, xDECIDE analyzes medical records and generates a ranked list of treatment options. Human experts then review these options, providing personalized treatment recommendations that enhance the precision and effectiveness of cancer care (Source).
Color Genomics
The prospect of affordable, comprehensive genetic testing is no longer a distant dream, thanks to Color Genomics. This innovative startup has revolutionized genetic testing by offering hereditary cancer risk assessments that are both affordable and thorough. By analyzing specific genes associated with cancer susceptibility, Color Genomics provides actionable insights to individuals and healthcare providers. This empowers patients to make well-informed decisions about preventive measures, such as undergoing prophylactic surgery or engaging in more frequent cancer screenings, thereby significantly improving their health outcomes (Source).
23andMe
Known for its direct-to-consumer genetic testing, 23andMe goes beyond ancestry reports to provide health-related insights. It offers crucial information on carrier status for various conditions and predispositions to diseases. For instance, if a customer discovers an increased risk for late-onset Alzheimer’s disease, they can proactively adopt lifestyle changes or participate in clinical trials to mitigate the risk. This kind of personalized health insight makes significant strides in preventive medicine, enabling individuals to take control of their health and future (Source).
Impact of ML on Patient Outcomes and Healthcare Efficiency
These success stories highlight the transformative potential of machine learning in personalized medicine, offering more precise, efficient, and effective healthcare solutions tailored to individual patient needs. By leveraging machine learning, healthcare providers can offer more accurate diagnostics, personalized treatment plans, and proactive patient monitoring, resulting in improved patient outcomes and operational efficiencies.
Machine learning not only improves the quality of care but also makes healthcare more accessible and affordable. By automating routine tasks and optimizing resource allocation, ML solutions help healthcare organizations focus on what they do best: caring for patients. The success stories of xCures, Color Genomics, and 23andMe are proof that the integration of AI and ML in healthcare can bring about revolutionary changes, benefitting both healthcare providers and patients.
As we continue to innovate and apply machine learning in various aspects of healthcare, the future looks promising with enhanced precision in treatment, early disease detection, and overall improved healthcare delivery.
Conclusion
The integration of machine learning into the healthcare industry is more than a technological advancement—it’s a paradigm shift. By using machine learning algorithms, healthcare providers can make more accurate diagnoses, develop personalized treatment plans, and improve patient outcomes. The profound impact of machine learning and deep learning can be seen across various healthcare applications, from image recognition in radiology to predictive analytics in disease diagnosis.
Machine learning for healthcare opens up new possibilities, making it possible to identify diseases earlier and develop more effective treatments. The use of machine learning-based approaches, such as deep learning algorithms and supervised learning techniques, allows healthcare organizations to deliver more precise and tailored care. These advancements in healthcare not only elevate the quality of patient care but also enhance operational efficiencies, thereby reducing costs and optimizing resource allocation.
But it’s not just about the algorithms and the technology. Behind every successful machine learning system is a team of dedicated machine learning engineers and healthcare professionals who work tirelessly to fine-tune these models. Their efforts ensure that the AI in healthcare remains accurate, ethical, and beneficial to all patients.
The application of machine learning models in healthcare also paves the way for groundbreaking medical research. Machine learning can be used to analyze vast datasets, uncovering patterns and correlations that were previously hidden. This enables researchers to predict disease trends, develop new treatments, and even prevent outbreaks before they occur. The future of machine learning in healthcare looks promising, with numerous potential use cases that can revolutionize the field.
Moreover, the advantages of machine learning are not confined to clinical settings. Machine learning solutions can automate administrative tasks, streamline hospital operations, and improve access to healthcare services. By using machine learning classifiers and other advanced techniques, healthcare providers can make informed decisions quickly and efficiently.
However, as we embrace these technologies, it’s essential to remain aware of the potential risks of machine learning. Ethical considerations and data privacy must be at the forefront of any healthcare application, ensuring that patient information is secure and used responsibly.
In summary, the incorporation of artificial intelligence and machine learning in healthcare is transforming how we approach medicine and patient care. The combined power of AI and machine learning technologies offers unparalleled opportunities for innovation, making healthcare more efficient, accessible, and personalized. By continuing to explore and adopt these advancements, healthcare professionals can ensure that the benefits of machine learning are realized, paving the way for a healthier future for all.