Machine Learning: Business Use Cases You Need to Know
Introduction
Have you ever wondered how modern businesses manage to keep up with the rapidly changing market demands, while also improving efficiency and productivity? The answer often lies in the powerful capabilities of machine learning and artificial intelligence (AI). Imagine a world where mundane tasks are automated, customer experiences are tailored just for you, and financial risks are minimized with pinpoint accuracy. It might sound like science fiction, but for many businesses, this is today’s reality, thanks to the adoption of machine learning models and AI.
In recent years, the adoption of machine learning in business has soared, with approximately 49% of organizations leveraging AI to enhance revenue and market share. This transformative technology isn’t just for tech giants; it’s being embraced across various business sectors, from finance to customer service, and everything in between. Companies are increasingly investing in machine learning applications because of their potential to revolutionize traditional business processes and usher in a new era of efficiency, accuracy, and innovation.
In this article, we will delve into the game-changing use cases of machine learning for business. We will explore how this subfield of artificial intelligence is being used to enhance operational efficiency, engage customers, provide financial insights, manage workforce, optimize supply chains, and fuel innovation. By understanding the vast potential and benefits of machine learning, business leaders can make informed decisions to stay ahead in the competitive landscape.
Let’s embark on this journey to uncover the secrets of machine learning and its profound impact on business operations.
Enhancing Operational Efficiency
Think of machine learning (ML) as the unsung hero working tirelessly in the background, automating mundane tasks and optimizing processes to drive efficiency. For many modern businesses, ML is the silent engine ensuring that everything runs smoothly. This transformative technology is being widely adopted across various industries, reflecting its profound impact on enhancing business operations.
Real-Time Applications of ML in Business Operations
Predictive Maintenance in Manufacturing
- Machine learning algorithms are applied to predictive maintenance in manufacturing, where they analyze data from machines to predict potential failures before they occur. This proactive approach not only reduces downtime but also extends the lifespan of the equipment. Manufacturing businesses that use ML can ensure smooth operations and significant cost savings.
Automation of Repetitive Tasks
- Many business processes involve repetitive tasks that can be automated using machine learning. For instance, ML models can handle data entry, categorizing emails, and automating customer queries. This frees up valuable time for employees, allowing them to focus on more strategic initiatives.
Statistical Backing: Adoption and Impact
- Adoption Rate: Approximately 49% of organizations are utilizing machine learning and artificial intelligence to enhance revenue and market share, particularly in marketing and sales processes (Hollywood Gazette, Intuition).
- Investment in AI: A substantial 91.5% of businesses are increasing their investments in AI technologies, indicating a strong commitment to integrating these capabilities into their operations (Hollywood Gazette).
- Productivity Gains: Companies that have implemented AI report productivity improvements, with estimates suggesting a potential increase of up to 54% (Finances Online).
Benefits for Businesses
- Increased Efficiency: By automating mundane and repetitive tasks, machine learning enables businesses to operate more efficiently. ML algorithms process data at unparalleled speeds, providing real-time insights that can inform business decisions.
- Cost Savings: The predictive capabilities of machine learning reduce operational costs by minimizing downtime and optimizing resource allocation.
- Employee Productivity: Automating routine tasks allows employees to focus on high-value activities, thereby boosting overall productivity and job satisfaction.
Real World Example: Predictive Maintenance at General Electric (GE)
General Electric (GE) has been at the forefront of using machine learning for predictive maintenance. GE uses ML models to analyze vast amounts of data from sensors on their machines, from jet engines to electric turbines. By predicting when maintenance is needed, GE ensures their machinery operates efficiently, reducing downtime and maintenance costs. This is a prime example of how understanding machine learning can revolutionize business operations.
Sources:
- Hollywood Gazette: Machine Learning Statistics
- Intuition: Machine Learning by the Numbers
- Finances Online: Machine Learning Statistics
Machine learning is proving to be an invaluable tool for businesses looking to streamline their operations. By adopting ML, companies can unleash new levels of operational efficiency and cost-effectiveness, paving the way for a more productive future.
Discover how our tailored machine learning solutions can help you revolutionize patient care and streamline operations.
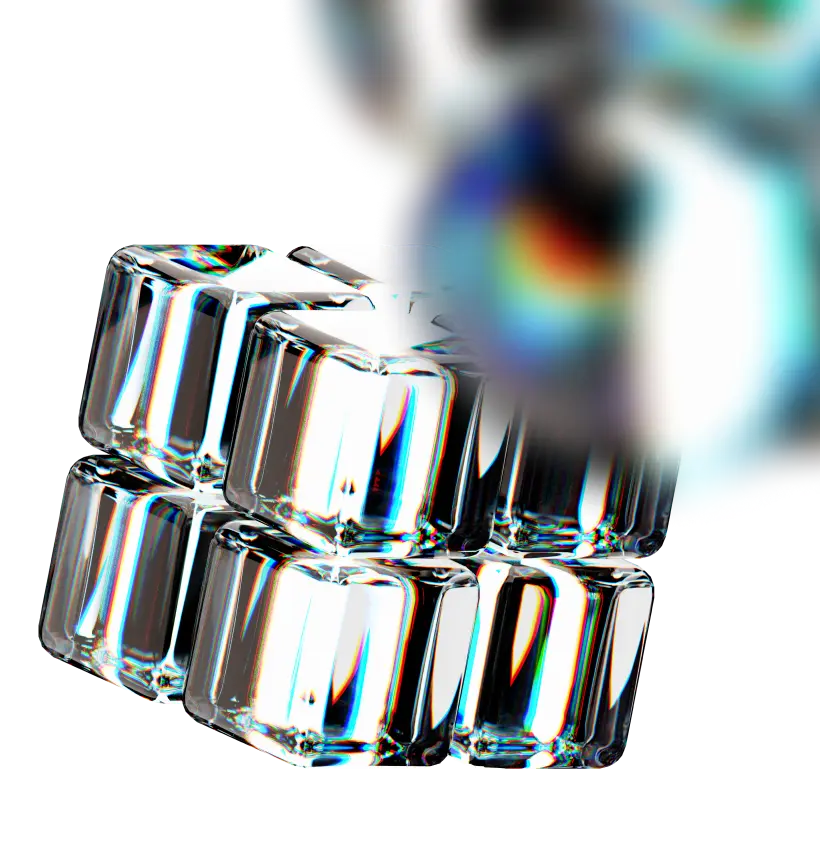
Customer Engagement
Ever noticed how online stores seem to know exactly what you want? That’s not just a lucky guess—it’s machine learning at work. By analyzing vast amounts of customer data, ML algorithms can identify patterns in data to offer personalized recommendations, improving the overall shopping experience. It’s like having a personal shopper who never sleeps, constantly learning from data to predict your preferences.
Key Applications of Machine Learning in Customer Engagement
Personalized Recommendations
- Machine learning algorithms analyze your browsing history, purchase history, and even social media data to tailor product recommendations. This personalization increases the likelihood of a purchase, enhancing customer satisfaction and loyalty. For example, Amazon’s recommendation engine uses machine learning to suggest products based on customer behavior.
Customer Support Automation
- Chatbots powered by AI and ML can handle customer queries efficiently, providing instant responses. These bots learn from each interaction, improving their responses over time. This not only enhances customer experience but also reduces the workload on human support agents.
Sentiment Analysis
- By using natural language processing, a subfield of artificial intelligence, businesses can analyze customer reviews and feedback. This helps in understanding customer sentiment, enabling businesses to make informed decisions to improve products and services.
Statistical Insights: Importance of Customer Experience
- Top-Use Case: Over half of the businesses (57%) use machine learning to improve customer experience, making it the top use case for the technology (AIPRM, Intuition).
- Fraud Detection: The financial sector, one of the largest adopters of ML, uses it for customer interaction, performance analysis, and fraud detection (AIPRM).
Sources:
Benefits for Businesses
- Enhanced Customer Loyalty: Personalized experiences make customers feel valued, increasing their loyalty to the brand.
- Increased Sales: Targeted recommendations are more likely to result in purchases, boosting sales figures.
- Efficient Customer Support: Automated systems can handle common queries, allowing human agents to focus on more complex issues.
Real World Example: Netflix’s Recommendation System
Netflix has set a gold standard for personalized customer engagement using machine learning. Their recommendation system uses deep learning algorithms to analyze viewing history and preferences. By understanding what customers like to watch, Netflix provides personalized suggestions that keep viewers engaged, thereby reducing churn and increasing subscription rates.
Machine learning continues to redefine customer engagement strategies, turning casual browsers into loyal customers. By leveraging ML, businesses can offer a more personalized, efficient, and rewarding shopping experience, driving both customer satisfaction and revenue growth.
Financial Insights
Navigating the financial landscape is like sailing through turbulent waters—full of risks and opportunities. In today’s business environment, machine learning (ML) and artificial intelligence (AI) serve as the navigational tools helping organizations steer clear of hazards while identifying lucrative pathways.
How Machine Learning Transforms Finance
Fraud Detection: The Digital Detective 🔍 Traditional fraud detection methods often felt like searching for a needle in a haystack. However, machine learning changes the game by analyzing patterns in vast amounts of data. Algorithms learn from transactional data to detect anomalies that may signify fraud, much like a detective spotting subtle clues. This proactive approach significantly reduces the risk of financial fraud.
Predictive Analytics for Risk Management 📉 In finance, understanding risks is crucial. Machine learning models use historical data to generate predictive insights, helping businesses identify future financial risks and opportunities. This leads to more accurate forecasting and better decision-making. Imagine having a crystal ball that provides insights based on data science rather than mysticism.
Algorithmic Trading: Speed and Precision 📈 Traders traditionally relied on gut feeling and experience, but now they have machine learning algorithms analyzing real-time data to make split-second trading decisions. These algorithms process vast amounts of data, identifying trends and executing trades at the most opportune moments. This application of machine learning not only boosts efficiency but also profitability.
Eye-Opening Statistics
- Investment Surge: A whopping 91.5% of businesses are ramping up their investments in AI and machine learning technologies (Hollywood Gazette).
- Productivity Boost: Companies using AI report productivity improvements, with increases up to 54% (Finances Online).
Sources:
Case Study: JPMorgan Chase
JPMorgan Chase has been a pioneer in adopting machine learning and AI to streamline their financial operations. Their COiN (Contract Intelligence) platform uses natural language processing (NLP) to analyze legal documents. This advanced tool can review thousands of documents in seconds, extracting key data points with remarkable accuracy. The result? Substantial time and cost savings, bolstering the bank’s bottom line.
Benefits Galore
- Enhanced Accuracy: Machine learning delivers highly accurate financial forecasts, allowing businesses to make informed decisions.
- Improved Security: Enhanced fraud detection algorithms help protect sensitive financial data, offering peace of mind.
- Operational Efficiency: Automating routine tasks with machine learning tools means human resources can focus on strategic, high-value activities.
Data Science Techniques in Finance
- Supervised Learning: This technique is key for tasks like fraud detection and credit scoring. It uses labeled training data to make accurate predictions.
- Unsupervised Learning Algorithms: Ideal for identifying unexpected patterns or anomalies in data sets, unsupervised learning is useful for complex, dynamic financial landscapes.
- Reinforcement Learning: This adaptive learning technique is particularly effective in algorithmic trading, where it helps optimize trading strategies.
Machine learning and AI are revolutionizing the financial sector, making it more secure, efficient, and data-driven. By deploying advanced analytics and predictive tools, today’s businesses can navigate the complex financial landscape with greater confidence and precision.
Workforce Management
Imagine a recruitment process where finding the perfect candidate is as easy as clicking a button. Machine learning models not only streamline hiring but transform the entire workforce management ecosystem. By leveraging AI and machine learning, companies can optimize talent acquisition, retention, and employee satisfaction.
The Power of Machine Learning in Workforce Management
Automated Resume Screening
- The Challenge: Sifting through hundreds of resumes manually is time-consuming and prone to bias.
- The Solution: Machine learning algorithms can filter and rank resumes based on predefined criteria, such as skill sets, experience, and education. This ensures that only the most suitable candidates reach the interview stage.
Predicting Employee Success
- ML models analyze historical data on successful hires to predict which candidates are most likely to excel at a company. This predictive analytics approach helps businesses make data-driven hiring decisions, improving long-term employee success rates.
Retention Strategies
- High employee turnover can be a significant drain on resources. Machine learning can identify patterns in employee behavior that may indicate dissatisfaction, allowing HR to intervene proactively. For example, analyzing interactions, feedback, and performance reviews can provide insights into the factors contributing to employee attrition.
Tangible Benefits
- Efficiency: Automating the initial stages of recruitment saves invaluable time.
- Diversity and Inclusion: AI can help eliminate unconscious biases in the hiring process, promoting a more inclusive workforce.
- Employee Satisfaction: Proactive retention strategies result in happier, more engaged employees.
Real-World Example: Unilever
Unilever has been using AI and machine learning tools to revolutionize its hiring process. The company employs algorithms to evaluate video interviews, assessing candidates based on their tone, word choice, and facial expressions. This technology allows Unilever to make objective, data-driven decisions, enhancing the quality of hires while reducing the time needed to fill positions.
Behind the Technology
- Supervised Machine Learning: Used extensively for resume screening and predictive analytics in workforce management.
- Natural Language Processing (NLP): This subset of AI is crucial for analyzing candidate interactions during interviews and performance reviews.
- Training Data: High-quality training data is the backbone of effective machine learning models. For workforce management, this involves aggregating vast amounts of employee-related data to train the algorithms accurately.
Did you know?
- Around 51% of companies are early adopters of machine learning technology, integrating it into various business processes like hiring and employee retention (Finances Online).
- By increasing their investment in AI and machine learning, 91.5% of businesses show a strong commitment to incorporating these technologies (Hollywood Gazette).
Machine learning is a strategic advisor enabling companies to make informed, data-driven decisions in workforce management.
Supply Chain Optimization
Optimizing supply chains can often feel like solving a complex puzzle with too many moving parts. Machine learning (ML) steps in as the ultimate problem-solver, transforming traditional logistics and supply chain management practices. By leveraging predictive analytics and vast amounts of data, ML enables businesses to streamline their supply chains, ensuring products move efficiently from A to B.
Key Applications of Machine Learning in Supply Chain
Demand Forecasting:
- Overview: Machine learning algorithms analyze historical sales data, customer trends, and market conditions to predict future demand.
- Benefit: Accurate demand forecasting helps in maintaining optimal inventory levels, reducing both overstock and stockouts.
Optimizing Inventory Management:
- Overview: ML models can determine the right amount of stock to hold and the best times to reorder.
- Benefit: This reduces storage costs and improves cash flow while ensuring product availability.
Enhancing Logistics & Delivery:
- Overview: Machine learning can optimize delivery routes and schedules by analyzing traffic patterns, weather conditions, and delivery windows.
- Benefit: Improved delivery accuracy and reduced transportation costs result.
Statistical Insights: The Value of Machine Learning
- Investment in Technology: A significant 91.5% of businesses are increasing their investments in AI and machine learning, underlining the importance of these technologies for operational efficiency (Hollywood Gazette).
- Market Growth: The global machine learning market is projected to be worth over $500 billion by 2030, driven by a compound annual growth rate (CAGR) of approximately 38.1% (Intuition).
Pioneering Example: Amazon
Amazon uses machine learning to optimize various aspects of its supply chain. From predicting customer demand to automating warehouse operations with robotics, Amazon’s use of ML ensures that orders are fulfilled quickly and efficiently. Their machine learning models analyze data points like customer preferences and seasonal trends, enabling the company to maintain an agile supply chain.
The Technology Behind It
- Predictive Analytics: By using historical and real-time data, predictive analytics provides actionable insights that drive supply chain decisions.
- Reinforcement Learning: This ML technique helps in optimizing delivery routes and warehouse operations by learning from past decisions and continuously improving.
- Data Science: Data scientists play a crucial role in developing and refining machine learning models that analyze patterns in the data to make accurate predictions.
Benefits for Businesses
- Improved Customer Satisfaction: Timely product availability and efficient delivery enhance the overall customer experience.
- Cost Efficiency: Optimized inventory and logistics reduce operational costs, boosting the bottom line.
- Agility: Machine learning enables businesses to quickly adapt to market changes, ensuring a resilient supply chain.
A Fact Worth Mentioning
More than half of the businesses (57%) use machine learning to enhance customer experience, according to AIPRM. This often includes applications in supply chain optimization, where efficient logistics directly influence customer satisfaction.
Machine learning has become the logistical mastermind behind seamless business operations. By applying machine learning techniques to enhance supply chain management, businesses can achieve unprecedented levels of efficiency, accuracy, and customer satisfaction.
Innovation and Product Development
Staying ahead often means continuously innovating and developing new products. Here, machine learning (ML) emerges as the secret weapon that provides the edge. By accelerating research and development (R&D) and identifying market opportunities, ML empowers companies to lead, rather than follow.
Accelerating R&D with Machine Learning
Faster Prototyping:
- Overview: Machine learning algorithms can simulate and test prototypes much faster than traditional methods.
- Benefit: This speeds up the development cycle, allowing companies to bring products to market more quickly.
Predictive Analytics for Market Trends:
- Overview: ML models analyze vast amounts of market data, including social media data and customer reviews, to predict future trends.
- Benefit: Companies can better align their product development strategies with market demands, ensuring higher success rates.
Innovation Identification:
- Overview: Machine learning can sift through big data to identify unmet needs and potential innovation opportunities in the market.
- Benefit: This proactive approach helps businesses stay ahead of competitors by launching unique, high-demand products.
Real World Example: Tesla
Tesla uses machine learning extensively in its product development process. From autopilot features that use reinforcement learning to improve driving algorithms, to analyzing new design concepts with predictive analytics, Tesla ensures its products are at the cutting edge of technology. This has positioned Tesla as a leader in the electric vehicle market, consistently innovating and setting new standards.
The Role of Data Scientists
Data scientists are crucial in extracting valuable insights from data sets and training data. They use various machine learning algorithms, from supervised to unsupervised learning, to create models that drive innovation. Here’s how:
- Supervised Learning: Used to predict outcomes based on historical data, such as customer preferences.
- Unsupervised Machine Learning: Identifies patterns and relationships within data sets without predefined labels, useful for discovering hidden opportunities.
- Natural Language Processing: Applied in analyzing customer feedback and market sentiment to guide product improvements.
Top Benefits of ML in Product Development
- Reduced Time-to-Market: Accelerated R&D cycles thanks to predictive analytics and rapid prototyping.
- Enhanced Product Quality: Continuous learning from data leads to iterative improvements, resulting in superior products.
- Market Alignment: Data-driven insights ensure that new products meet actual market needs and preferences.
Industry Insights
- A significant portion of companies, around 51%, are early adopters of ML technology, integrating it into their product development processes (Finances Online).
- The potential economic impact is enormous, with AI and ML expected to increase global GDP by 14% by 2030, demonstrating their transformative power in driving innovation (AIPRM).
Machine learning takes on the role of an essential tool in product development, enabling companies to innovate rapidly and effectively. By leveraging ML capabilities, businesses can transform data into actionable insights, leading to groundbreaking products and a significant competitive edge.
Conclusion
Machine learning has emerged as a game-changer. From enhancing operational efficiency to revolutionizing product development, the applications of machine learning in business are vast and varied. Companies that embrace machine learning and AI can unlock new levels of efficiency, customer satisfaction, and innovation.
Key takeaways from our exploration include:
- Operational Efficiency: Machine learning automates mundane tasks and optimizes business processes, resulting in substantial productivity gains and cost savings.
- Customer Engagement: Personalized recommendations and automated customer support enhance the customer experience, driving loyalty and sales.
- Financial Insights: ML provides accurate financial forecasts and fraud detection, enabling businesses to navigate financial complexities with confidence.
- Workforce Management: Automated resume screening and predictive analytics improve recruitment and retention, promoting a more efficient and diverse workforce.
- Supply Chain Optimization: Predictive analytics and reinforcement learning streamline logistics and inventory management, ensuring timely delivery and cost efficiency.
- Innovation and Product Development: Accelerated R&D and data-driven insights lead to market-aligned, high-quality products.
Machine learning is a strategic asset that empowers businesses to make data-driven decisions, innovate swiftly, and stay ahead of the competition. Those who understand and implement these technologies will be well-positioned for success in the rapidly evolving business world.
The future is now, and machine learning is paving the way.