Unlocking Business Analytics: Machine Learning in Business Applications
Picture a bustling company, decisions flying left and right, and in the middle of it all, a calm, calculated voice providing insights that make every move a strategic masterstroke. That voice? It’s powered by machine learning (ML). How often have you wished your business could predict market trends as accurately as a seasoned weather forecaster predicts the coming storm?
Welcome to the future of business analytics, where machine learning is revolutionizing the way companies analyze data, make predictions, and drive growth. Imagine having the power to turn chaos into clarity with just a few clicks – leveraging vast amounts of data to glean valuable insights and make informed, data-driven decisions. This article dives into the profound impact of machine learning in business analytics, exploring how machine learning models, algorithms, and AI are transforming the business landscape.
The Power of Predictive Analytics
Imagine having the power to see into the future. That’s what predictive analytics offers. Leveraging machine learning algorithms, businesses can analyze historical data and derive accurate predictions, allowing them to stay ahead of the curve. From predicting customer churn to forecasting sales trends and market movements, predictive analytics is akin to having a crystal ball tailored for the business world.
The Power of Predictive Analytics: A Game-Changer
Forecasting Future Trends: Machine learning models analyze vast amounts of historical data to identify patterns and trends, enabling businesses to make predictions or decisions based on data-driven insights. For example, a retail company can leverage machine learning to forecast product demand, helping them optimize inventory management and avoid stockouts or overstock situations.
Improving Marketing Strategies: Predictive analytics aids in tailoring marketing efforts more effectively. By understanding customer behavior and preferences, businesses can create personalized marketing strategies that resonate with their target audience, ultimately boosting their marketing ROI by 15-20% [5].
Enhancing Risk Management: Whether it’s in finance, healthcare, or retail, predictive analytics can identify potential risks and anomalies. Financial institutions, for instance, can leverage machine learning for fraud detection, saving millions in potential losses by detecting suspicious transactions in real-time.
Boosting Customer Retention: By analyzing customer data, businesses can predict which customers are likely to churn and implement targeted interventions to retain them. This proactive approach not only fosters customer loyalty but also drives long-term growth.
Market Growth and Adoption
Market Size: The global machine learning market was valued at $15.44 billion in 2021, with projections to skyrocket to $209.91 billion by 2029, reflecting a compound annual growth rate (CAGR) of 38.8% [4].
Business Intelligence Software: The business intelligence and analytics software market is expected to grow at a CAGR of 9.6% from 2020 to 2027, anticipated to reach $55.48 billion by 2027 [3].
These statistics emphasize the escalating role of machine learning in business analytics, marking it as a critical component in the evolving business landscape. Implementing machine learning into business processes not only facilitates real-time data analysis but also fosters data-driven decision-making, giving businesses a competitive edge.
Customer Insights: Getting to Know Your Customers
In the age of data, understanding your customers is crucial. Machine learning in business dives deep into customer data, unveiling patterns and preferences that would be impossible to detect manually. It’s like having a secret dossier on each customer, allowing businesses to tailor experiences, improve satisfaction, and build loyalty. Machine learning applications such as customer segmentation and personalized marketing are helping businesses revolutionize their customer engagement strategies [2][5].
How Machine Learning Helps Understand Customers
Customer Segmentation: Using machine learning algorithms, businesses can segment their customer base into distinct groups based on purchasing behavior, preferences, and demographics. This segmentation allows for more personalized marketing strategies and tailored marketing efforts.
Sentiment Analysis: Machine learning techniques, particularly natural language processing (NLP), enable businesses to analyze customer reviews and social media interactions. Through sentiment analysis, companies can gauge public sentiment towards their products and services, making informed decisions to enhance customer experiences.
Predictive Customer Behavior: By analyzing historical data, machine learning models can predict future customer behavior. Whether it’s predicting which products a customer is likely to purchase next or identifying potential churn risks, these insights empower businesses to take proactive measures.
Benefits of Customer Insights
Personalized Marketing Campaigns: With accurate predictions and segmentation, businesses can deliver personalized marketing that resonates with individual customers, thereby improving engagement and conversion rates.
Enhanced Customer Retention: By identifying at-risk customers, businesses can implement targeted retention strategies, reducing churn and fostering long-term loyalty.
Improved Customer Satisfaction: Tailoring customer experiences based on insights derived from machine learning leads to higher customer satisfaction and positive brand perception.
Examples of Machine Learning in Customer Insights
Application | Description | Benefits |
---|---|---|
Customer Segmentation | Dividing customers into different groups based on behavior and demographics | Personalized marketing, targeted campaigns |
Sentiment Analysis | Using NLP to analyze customer feedback and social media interactions | Understanding public sentiment, improving product features based on feedback |
Behavior Prediction | Analyzing past behaviors to predict future actions | Proactive customer engagement, risk management |
By integrating machine learning into customer data analysis, businesses can harness the power of AI to draw valuable insights from vast amounts of data. This not only enables more informed decision-making but also adds a competitive edge in the modern business landscape.
Using machine learning models to analyze data and make real-time data-driven decisions can propel businesses ahead of their competition. As machine learning continues to evolve, the insights it provides will become even more refined and actionable, driving substantial growth and innovation across various sectors.
Explore how our expert solutions can revolutionize your operations and drive innovation in your company.
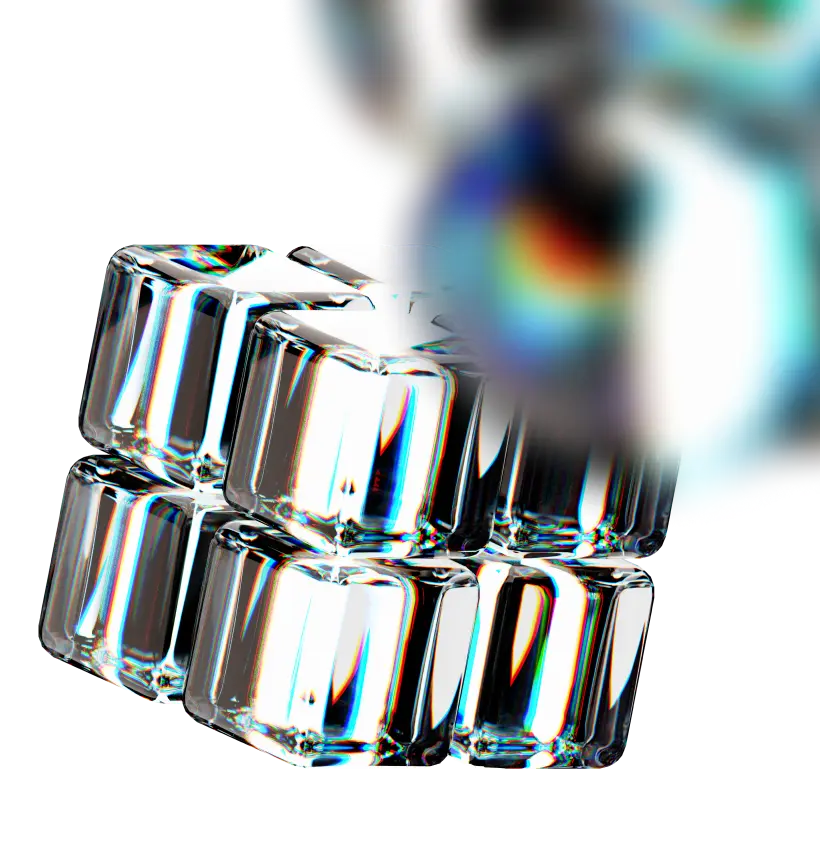
Operational Efficiency: The Silent Optimizer
Behind every successful operation is a myriad of processes, each ripe for optimization. Machine learning in business identifies inefficiencies and suggests improvements, from supply chain logistics to inventory management. Think of it as a tireless analyst, constantly finding ways to streamline operations and cut costs. The ability to automate and accelerate data analysis processes has led to significant operational efficiencies [1].
Enhancing Operational Efficiency with Machine Learning
Supply Chain Optimization: Supply chain operations are complex and multifaceted. Machine learning models can analyze vast amounts of data from various sources to identify patterns and trends, making forecasts that optimize logistics, reduce waste, and improve overall efficiency.
Inventory Management: Accurate inventory management is crucial for reducing costs and meeting customer demand. Machine learning algorithms help businesses predict inventory needs based on historical data and market trends, ensuring that the right products are available at the right time.
Process Automation: From detecting anomalies to automating routine tasks, machine learning aids in improving business processes. It reduces the manual workload and allows human analysts to focus on more strategic tasks, ultimately driving higher productivity.
Benefits of Operational Efficiency
Cost Reduction: By identifying inefficiencies, machine learning helps businesses reduce operational costs, from minimizing waste in the supply chain to optimizing resource allocation.
Improved Decision-Making: Real-time data analysis and machine learning insights enable businesses to make informed decisions quickly, adapting to changes and mitigating risks effectively.
Enhanced Productivity: Automating repetitive tasks and optimizing processes frees up valuable time for employees, allowing them to focus on innovation and strategic initiatives.
Examples of Machine Learning in Operational Efficiency
Application | Description | Benefits |
---|---|---|
Supply Chain Optimization | Analyzing logistics data to optimize routes, reduce delivery times, and manage inventory | Reduced costs, improved delivery performance |
Inventory Management | Forecasting inventory needs based on historical sales data and market trends | Lower inventory costs, better stock availability |
Process Automation | Automating routine tasks like data entry, anomaly detection, and customer inquiries | Increased productivity, fewer errors |
By leveraging machine learning techniques, businesses can transform their operational functions, leading to significant improvements in efficiency and effectiveness. Machine learning helps in analyzing vast amounts of data to make predictions or decisions, providing companies with the competitive edge needed in today’s fast-paced business environment.
Operational efficiency also plays a critical role in improving customer satisfaction and driving business growth. The integration of machine learning into business processes represents a major shift toward smarter, data-driven decision-making.
Financial Forecasting
Steering a company’s finances is no easy task, but machine learning in business makes it a lot smoother. By analyzing financial data, machine learning models provide accurate forecasts, helping businesses make informed budgeting, investment, and growth decisions. Imagine having a seasoned financial advisor on your team, 24/7.
Transforming Financial Management with Machine Learning
Machine learning plays a pivotal role in financial forecasting by leveraging historical data and real-time analytics. Here’s how:
Precision in Budgeting Traditional budgeting often involves guesswork. Machine learning algorithms analyze historical spending data and market conditions to create precise budgeting forecasts. This reduces the margin of error and ensures that resources are allocated efficiently.
Astute Investment Choices Investing is another arena where machine learning has profound implications. By processing vast amounts of historical and real-time data, machine learning models predict market trends and assess the viability of various investment options. This capability facilitates informed investment decisions and optimizes returns.
Proactive Risk Management Financial risks are inherent in any business operation. Machine learning aids in preemptively identifying potential risks by analyzing patterns and anomalies in financial data. This proactive approach helps in mitigating risks before they can impact the company’s financial health.
Real-World Success Story
Take the example of Company X, a financial services firm that integrated machine learning into their financial forecasting. Previously reliant on traditional analytics methods, they faced frequent budgeting discrepancies and missed investment opportunities. After implementing machine learning algorithms, they witnessed a 20% improvement in budget accuracy and a significant reduction in financial waste. Moreover, their investment portfolio grew by 15% within a year, thanks to AI-driven insights.
The Benefits: Why Businesses Should Embrace Machine Learning
Accurate Predictions: Machine learning models continuously learn and adapt, providing highly accurate financial forecasts that reflect current market conditions.
Data-Driven Decision Making: By transforming raw data into valuable insights, machine learning empowers businesses to make informed decisions, minimizing risks and maximizing returns.
Increased Agility: Real-time data analysis allows businesses to swiftly react to market fluctuations, making dynamic adjustments to their financial strategies.
“By 2021, organizations using AI and machine learning in business intelligence were expected to realize an additional $1.1 trillion in value” [3].
The Road Ahead
The future of business analysis looks increasingly data-driven. By leveraging the capabilities of artificial intelligence and machine learning in business analytics, companies can navigate the complexities of the financial seas with confidence. Machine learning transforms the decision-making processes, ensuring sustainable growth and competitive advantage in an ever-evolving market landscape.
Usung machine learning for financial forecasting is a strategic move that redefines business outcomes and paves the way for a data-driven future.
Real-Time Analytics
Machine learning powers real-time analytics, offering businesses up-to-the-minute data on everything from website traffic to sales performance. Imagine having a live feed of your business’s health, allowing you to react instantly to any changes. According to reports, organizations that use AI and machine learning in business intelligence can realize an additional $1.1 trillion in value [3].
The Importance of Real-Time Analytics
Immediate Decision-Making: Real-time analytics enables businesses to make instantaneous, data-driven decisions. Whether it’s adjusting marketing strategies based on current customer interactions or managing supply chain operations more efficiently, the ability to act swiftly is crucial in a competitive market.
Enhanced Customer Experiences: By monitoring customer behavior in real-time, businesses can tailor their offerings to meet immediate customer needs. For example, e-commerce websites can adjust pricing strategies instantly based on real-time demand and customer sentiment.
Operational Agility: With real-time data, companies can quickly detect and rectify operational inefficiencies. For instance, a sudden drop in sales performance can be identified and addressed immediately, preventing potential revenue loss.
Real-World Applications of Real-Time Analytics
Application | Description | Benefits |
---|---|---|
Website Traffic Monitoring | Tracking visitor behavior in real-time to optimize user experience | Increased engagement, reduced bounce rates |
Sales Performance Analysis | Analyzing sales data as it happens to adjust marketing and sales strategies | Improved sales tactics, immediate issue resolution |
Supply Chain Management | Monitoring supply chain operations live to predict and solve potential disruptions | Enhanced efficiency, minimized delays and costs |
Key Benefits of Real-Time Analytics
Proactive Decision-Making: Real-time insights allow businesses to anticipate issues and opportunities, making proactive decisions that are based on the most current data.
Operational Efficiency: Continuous monitoring and instant data processing lead to more efficient business operations, reducing downtime and improving overall productivity.
Competitive Edge: In a highly competitive business landscape, the ability to react immediately to market changes can be a determining factor in maintaining and growing market share.
“With real-time analytics, we not only monitor our current performance but also anticipate future trends. It’s like having a constant pulse on our business health.” – Attract Group CEO, Vladimir Terekhov
Real-Time Analytics for a Business Environment
Real-time analytics, powered by machine learning and AI, is transforming how businesses operate. By providing immediate insights into various business functions, companies can react swiftly and efficiently, ensuring optimal performance and customer satisfaction. As the technology continues to evolve, the abilities to analyze vast amounts of data in real-time will become even more integral to business success.
Real Case Studies
Machine learning (ML) has become a pivotal tool in business operations management, offering innovative solutions across various industries. Here are some notable case studies that illustrate the application of ML in business operations:
1. Healthcare: Mercy Health System
Mercy, a large healthcare system, leveraged ML to modernize its data infrastructure and improve patient outcomes. By using Azure Machine Learning, Mercy integrated vast amounts of data to predict patient conditions and optimize care pathways. This approach significantly reduced hospital stays and enhanced patient care through personalized treatment plans (Source: Mercy Health System).
2. Human Resources: Randstad
Randstad, a global human resources provider, utilized ML to enhance its data analytics capabilities. By partnering with Google Cloud, Randstad developed a specialized CRM system named Signal, which improved sales outreach success from 25% to 70%. The ML model provided insights into the job market, allowing for more strategic customer engagement (Source: Randstad).
3. Pricing Strategy: IBM
IBM implemented ML to optimize its pricing strategies through the Cognitive Pricing Analytics system. This system analyzes historical transaction data and market trends to recommend optimal pricing for customers. The result was a 41% acceptance rate for generated quotes and a streamlined pricing approval process (Source: IBM).
4. Supply Chain Management
ML techniques are extensively applied in supply chain and operations management (OSCM) to handle big data and nonlinear problems. For instance, ML algorithms are used for supplier selection, quality control, and demand forecasting, enhancing decision-making and operational efficiency (Source: Supply Chain Management).
5. Retail and Customer Engagement: Starbucks
Starbucks uses ML to enhance customer engagement by delivering personalized offers and recommendations. By analyzing customer data, Starbucks tailors its marketing efforts to individual preferences, thereby increasing customer satisfaction and loyalty (Source: Starbucks).
Key Takeaways from These Case Studies
- Accuracy and Precision: Machine learning enables businesses to make accurate predictions and informed decisions across various functions.
- Operational Efficiency: By optimizing processes such as inventory management and supply chain operations, machine learning leads to significant cost savings and increased efficiency.
- Enhanced Customer Experiences: Personalized marketing and sentiment analysis help businesses understand and cater to customer needs more effectively.
- Proactive Risk Management: Real-time data analysis and anomaly detection enhance risk management and fraud prevention.
These case studies highlight the diverse applications of ML in business operations management, demonstrating its potential to drive efficiency, optimize strategies, and enhance customer experiences across various sectors.
Conclusion
Machine learning is revolutionizing business analytics, transforming vast amounts of data into actionable insights and giving companies a competitive edge. From predictive analytics to real-time data analysis, machine learning algorithms enable businesses to make informed, data-driven decisions. This profound impact spans various sectors, enhancing customer experiences, streamlining operations, and optimizing financial strategies.
The market growth for machine learning underscores its increasing adoption, with projections estimating the global market will reach $209.91 billion by 2029, reflecting a compound annual growth rate (CAGR) of 38.8% [4]. Companies leveraging the capabilities of machine learning models are not only driving substantial growth but are also gaining a competitive advantage in a highly dynamic business landscape [1][3].
However, the journey of integrating machine learning into business analytics is not without its challenges. Ensuring data quality, addressing privacy concerns, and overcoming the shortage of skilled data scientists are critical hurdles that businesses must navigate [1]. Despite these challenges, the potential benefits of machine learning far outweigh the obstacles, offering unprecedented opportunities for data-driven innovation and efficiency.
Organizations that embrace machine learning in their decision-making processes are better positioned to navigate the complexities of the modern market, making accurate predictions and optimizing their business functions. As the technology continues to evolve, machine learning will undoubtedly play an even more integral role in shaping the future of business analytics.